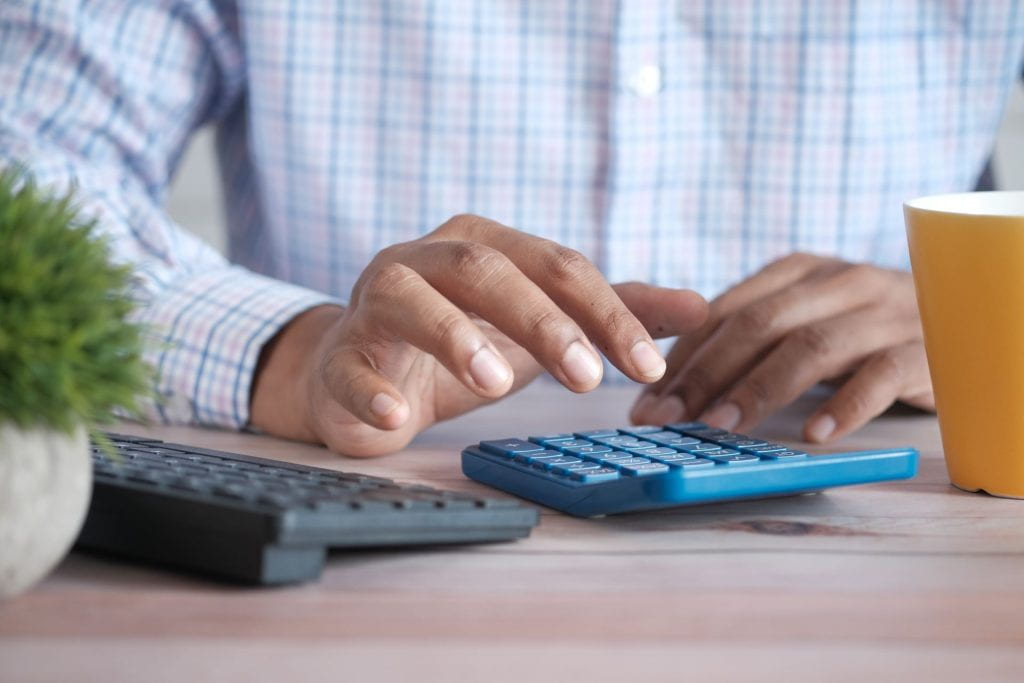
In recent years, clinical calculators have faced criticism for their treatment of race and ethnicity. The datasets on which these calculators were based, drawn from cohort studies and other longitudinal trials, are frequently homogeneous populations or of limited diversity. Reports of research methods have often been opaque. The data categories used for race and ethnicity, based on those created by the Office of Management and Budget, are limited and do not reflect the diversity of participants’ identities (see AMA Manual, 11th edition, Chapter 11.12.3). Furthermore, the data behind the calculators reflects existing disparities, which are perpetuated in their continued use (Vyas et al., 2020).
As the medical community confronts how the variable race serves a proxy for systemic racism in these calculations (Davidson et al., 2021), conversations have even risen to more mainstream media (e.g. Should Black People Get Race Adjustments In Kidney Medicine?, Racial bias in widely used hospital algorithm, study finds). As we reckon with the racism and discrimination that has been part of medicine; we advocate for, and work towards, change.
Time and research is needed to identify predictive variables, develop algorithms, and validate calculators (Hamad et al., 2022 video abstract, Rodriguez et al., 2019, Cardiovascular Risk Assessment [DynaMed]). For ASCVD risk estimation, for instance, we often use the Pooled Cohort Equations (PCE). The 2019 ACC/AHA Guideline on the Primary Prevention of Cardiovascular Disease notes, “The PCE are best validated among non-Hispanic whites and non-Hispanic blacks living in the United States. In other racial/ethnic groups or in some non-US populations, the PCE may overestimate or underestimate risk. Therefore, clinicians may consider the use of another risk prediction tool as an alternative to the PCE if the tool was validated in a population with characteristics similar to those of the evaluated patient” (Arnett et al., 2019, emphasis added). Fortunately, there are alternatives, which are listed in practice guidelines and in DynaMed, and work to develop and validate tools continues, e.g. Weale et al., 2021.
Over years, we see new calculators developed and guidelines begin to include them. More immediately, we see a statement on Race in Medical Calculators and Risk Estimates describing MDCalc’s efforts to provide additional context and signposting. Going back to ASCVD risk estimation, the ASCVD Risk Estimator+ now notes that “estimates may underestimate the 10-year and lifetime risk for persons from some race/ethnic groups, especially American Indians, some Asian Americans (e.g., of south Asian ancestry), and some Hispanics (e.g., Puerto Ricans), and may overestimate the risk for others, including some Asian Americans (e.g., of east Asian ancestry) and some Hispanics (e.g., Mexican Americans)”.
We can be advocates for changes. Medical students in a February 2022 informatics session questioned why one particular tool only had Black and White as options for race. (Other calculators offer Black | White | Other; the AMA manual of style notes that “The nonspecific group label "other"... is uninformative and may be considered pejorative" (AMA Manual of Style, Chapter 11.12.3).) Their librarian instructor contacted the tool developer, who responded by adding the option ‘Neither of these’. While the display changes are imperfect, they highlight the importance of continuing the discussion of how race is used in clinical calculators, and the importance of highlighting where we need to adopt additional tools, develop new tools, reconsider what we are trying to measure, and invite more people to plan and participate in our studies to ensure that we have data that reflects our population.
Research to improve these calculators continues. We have seen a reevaluation of the use of race as a variable at all in calculations like eGFR. Calculators are being developed and validated using data and variables that reflect more diverse populations. Researchers are being asked to consider how race as a social construct impacts their research questions, whether to use race as a variable, and, if so, what categories are appropriate. In the informatics session, we discuss how practitioners need to consider the data from which the calculators were derived, how that data does (not) reflect their patients, and what alternative tools they might use. Himmelfarb’s point-of-care tools highlight practice guidelines and recommended calculators. Our librarians are here to help you access and navigate these resources.
For more on this topic, see:
Awareness in Writing and Publishing, for information on collecting and reporting on race and ethnicity in research [Additional Resources - Cultural Competency]
Critical Data Literacy: Addressing Race as a Variable in a Preclinical Medical Education Session [poster; Research Guide]
Race Correction in the UTI Guidelines - The Curbsiders [podcast]
References
Vyas D. A., Eisenstein L. G., Jones D. S. Hidden in Plain Sight — Reconsidering the Use of Race Correction in Clinical Algorithms. The New England jJournal of medicine. 2020;383(9):874-882. https://doi.org/doi:10.1056/NEJMms2004740
Davidson, K. W., Krist, A. H., Tseng, C.-W., Simon, M., Doubeni, C. A., Kemper, A. R., Kubik, M., Ngo-Metzger, Q., Mills, J., & Borsky, A. (2021). Incorporation of Social Risk in US Preventive Services Task Force Recommendations and Identification of Key Challenges for Primary Care. JAMA. https://doi.org/10.1001/jama.2021.12833
Hamad, R., Glymour, M. M., Calmasini, C., Nguyen, T. T., Walter, S., & Rehkopf, D. H. (2022). Explaining the variance in cardiovascular disease risk factors: A comparison of demographic, socioeconomic, and genetic predictors. Epidemiology (Cambridge, Mass.), 33(1), 25–33. https://doi.org/10.1097/EDE.0000000000001425
Rodriguez, F., Chung, S., Blum, M. R., Coulet, A., Basu, S., & Palaniappan, L. P. (2019). Atherosclerotic cardiovascular disease risk prediction in disaggregated asian and hispanic subgroups using electronic health records. Journal of the American Heart Association, 8(14), e011874. https://doi.org/10.1161/JAHA.118.011874
Arnett D. K., Blumenthal R. S., Albert M. A., et al. 2019 ACC/AHA Guideline on the Primary Prevention of Cardiovascular Disease: A Report of the American College of Cardiology/American Heart Association Task Force on Clinical Practice Guidelines [published correction appears in Circulation. 2019 Sep 10;140(11):e649-e650] [published correction appears in Circulation. 2020 Jan 28;141(4):e60] [published correction appears in Circulation. 2020 Apr 21;141(16):e774]. Circulation. 2019;140(11):e596-e646. https://doi.org:/10.1161/CIR.0000000000000678
Weale, M. E., Riveros-Mckay, F., Selzam, S., Seth, P., Moore, R., Tarran, W. A., Gradovich, E., Giner-Delgado, C., Palmer, D., Wells, D., Saffari, A., Sivley, R. M., Lachapelle, A. S., Wand, H., Clarke, S. L., Knowles, J. W., O’Sullivan, J. W., Ashley, E. A., McVean, G., … Donnelly, P. (2021). Validation of an Integrated Risk Tool, Including Polygenic Risk Score, for Atherosclerotic Cardiovascular Disease in Multiple Ethnicities and Ancestries. The American Journal of Cardiology. https://doi.org/10.1016/j.amjcard.2021.02.032
AMA Manual of Style Committee. (2020). Correct and Preferred Usage. In AMA Manual of Style: A Guide for Authors and Editors (11th ed.). Oxford University Press. https://doi.org/10.1093/jama/9780190246556.001.0001